Back
Artificial Intelligence | 10 Concerns & 10 Responses
15min • 07 Oct 23
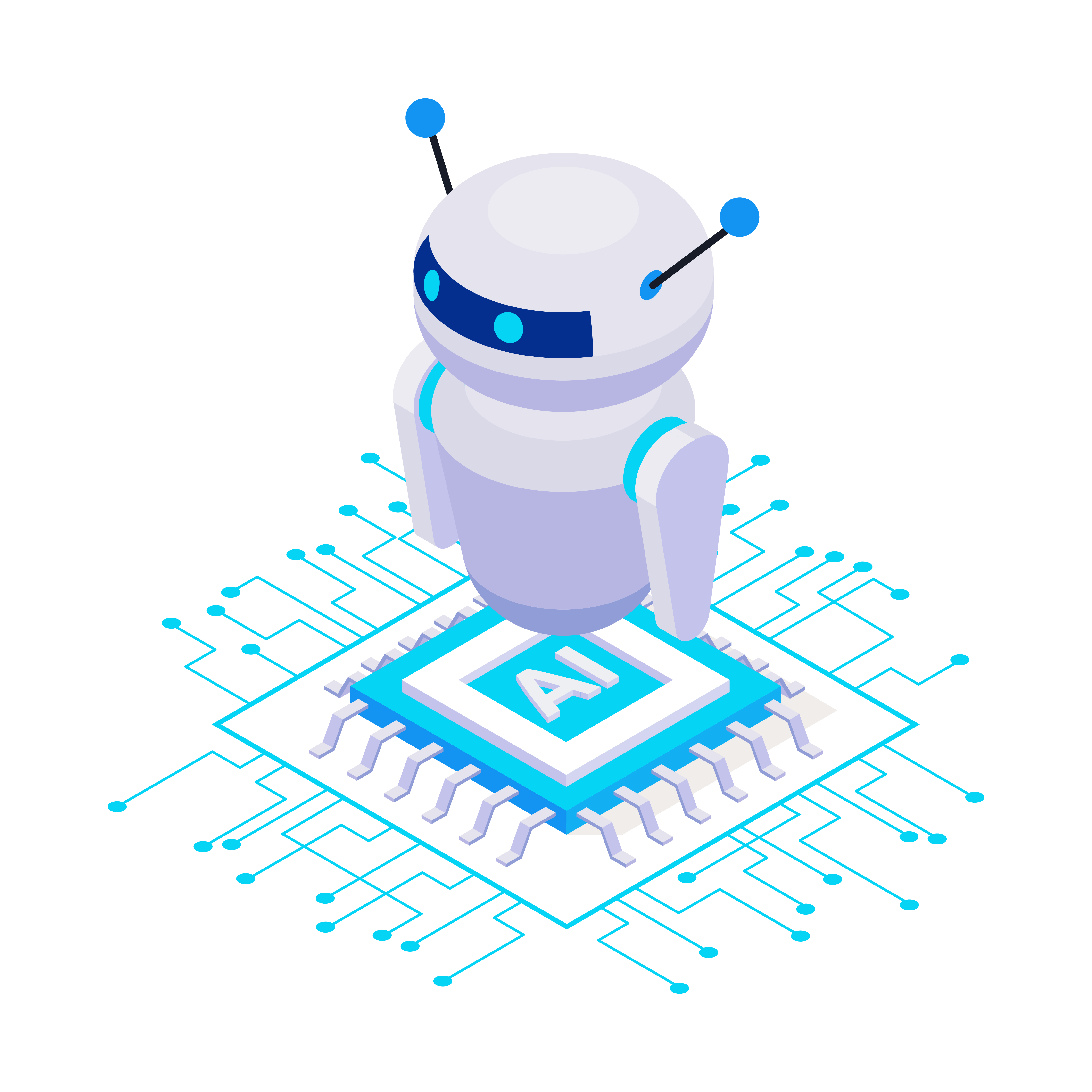
“As in-house teams, most of us are needing to find ways to do more with less. This is where AI creates immense opportunities.” - Bianca Lau (Head of Legal at Youi Insurance)
INTRODUCTION
Christopher Niesche's September 2021 survey of in-house lawyers ("IHL") reported an almost universal sentiment that:
the in-house legal profession was becoming increasingly complex as it assumes broader roles, but
in-house teams typically lack the “time, budget and understanding to adopt the legal technology tools that could help them.”
In this operational context, many in-house teams are hoping that AI will represent an easier tech-based pathway to achieve significant cost savings, especially for routine tasks that benefit from economies of scale. However, AI is a technology that represents certain reasonable concerns and dynamic challenges that the in-house community must be aware of and carefully manage.
Understanding the nature of AI technology usage and the many “as yet” unclear legal implications arising from its use will be increasingly important for the in-house community. It will require proactive consideration and on-going “horizon monitoring” by the in-house community if it is to be safely embraced in an ethical and compliant manner
So, in this Whitepaper, GLS seeks to speed up the process by which the in-house community can orientate its way around with the AI issue with a “heads up” briefing on::
• some of the key current issues with AI; and
• the emerging best practices for dealing with those issues.
Our hope is that the GLS Legal Operations Community will be better placed to more readily tap into the opportunities offered by AI in a safe, constructive and effective manner.
WHAT EXACTLY IS “AI”?
Artificial intelligence (“AI”) is the approximation of human intelligence processes by computer systems.
The term “AI” as currently used refers to specific AI applications including “expert systems”, “natural language processing”, “speech recognition” and “machine vision” and to certain components of the technology such as “machine learning” and “large-scale language models”.
Advanced forms of AI have been around for a surprisingly long time. The earliest successful AI program is generally accredited to Christopher Strachey’s 1951 checkers program. The AI based “tools” that successfully gained widescale utilisation were based on “discriminative” models. That is, they operated by learning the boundaries between various classes in a dataset.
- These discrimination AI tools made them excellent for classification problems (e.g. face vs tree, indemnity vs warranty etc).
Such AI has been incorporated into many very commonly used applications for years. For example, GLS LegalSifter as a contract review tool, and Adobe Photoshop’s range of “Auto-Select” tools are very different applications (for example GLS LegalSifter uses natural language processing) and use very different training data, but both are based on this discriminative model of AI.
AI’s recent prominence in the zeitgeist has occurred as a round of “Generative AI systems”, and in particular ChatGPT, have profoundly passed the “Turing Test”. These AI models have demonstrated exceptional performance, in the realm of natural language processing, such that the AI can now seemingly listen, learn, and even challenges human users at times.
OpenAI's ChatGPT tool is powered by large-scale language model trained on a massive amount of textual data. Such textual data was originally a “frozen” image of the internet from Sept 2021, but the latest editions of ChatGPT are to be connected to the Internet in real time.
- These generative AI tools means that a tool is now a creative, rather than an analytical force . (e.g. Prompt: “Draft a 10 word indemnity for the benefit of a customer” generates the response “Company indemnifies customer against any harm caused by company's negligence.”)
At the core of all of these AI models, is still however a “simple” process defined by a pattern of statistical relationships that exist between different features of data. These statistical relationships gain a dynamism and “life-like quality” as a result of machine learning’s ability to:
- to refine/evolve itself as it encounters new data; and
- to scale in response to HUGE datasets (e.g. the entire internet).
AI CONCERNS VS IHL RESPONSES
|
|
1. Privacy | 1. Privacy |
Regulators in Europe, and in particular Italy, are leading the way on this issue. Specifically, Italy’s data privacy regulator the “Garante” has flagged, amongst others, 3 key data privacy concerns with AI, and ChatGPT in particular:
In this context it is worth remembering that to use personal information an organisation must generally either i) obtain express consent from the data subjects (OpenAI didn’t do this), or ii) establish a “legitimate interest” to use the personal data. “Establishing a legitimate interest defence is very hard to do” - Lilian Edwards, Prof of Law, Innovation, and Society at Newcastle University OpenAI’s privacy policy states that it is relying on the “legitimate interests” theory, but it does not elaborate on what those interests actually are. The GPT-4 technical paper does note that its training data may include “publicly available personal information” and that OpenAI takes steps to protect people’s privacy, including “fine-tuning” models to stop people asking for personal information and removing people’s information from training data “where feasible.” It remains to be seen whether this will be sufficient for regulators. The issue facing AI developers is that just because someone’s information is public doesn’t mean that a developer can unilaterally decide to take it and use it for their own purposes. However, it is also unclear whether it is even possible for the existing AI tools to “forget” the personal information they have been trained on. Deleting something from an AI system that is inaccurate or objected to by a data subject is not the same as deleting a line item from a spreadsheet. This is particularly true as datasets and AI systems are rapidly being built “on-top” of each other, which makes it almost impossible to determine the origins of the data that is to be “deleted.” Edwards notes that it is currently not technically possible to uphold a data subject’s GDPR rights in relation to most existing AI systems: “There is no clue as to how you do that with these very large language models.. They [were not designed or built with] any provision for it.” | EU’s coordinated and well thought out approach to data privacy, means that it is typically the world's guiding force on privacy law. This will probably remain true for AI privacy issues. Shortly after the Garante announced its probe, regulators in France, Germany, Ireland and Norway all started to coordinate with the Garante with a view to initiating their own investigations. “If the business model has just been to scrape the internet for whatever you could find, then there might be a really significant issue here” - Tobias Judin (Head of International Date, Norway’s DP Authority) Data privacy is an issue that will get worked out eventually (probably sooner rather than later), but in the interim it’s best to use fake names and broad inquiries as much as possible.
![]()
|
2. Data Security | 2. Data Security |
AI represents 3 distinct problems for data security. Firstly, the network security of the AI developers themselves has already been breached in several well-reported incidents. For example, OpenAI confirmed a data breach of ChatGPT in May 2023. Secondly, Generative AI has proven itself to be both a powerful tool for businesses, but also for “bad actors.” At present, the key risk vectors appear to be:
Checkpoint Research recently demonstrated how, despite restricting themselves from writing any lines of code, easy it was to only use plain English prompts to:
While OpenAI has now implemented filters to stop the ChatGPT from generating phishing emails and malicious code, there are numerous ways to bypass those restrictions. For example, “WormGPT” is a readily available generative AI tool based on an earlier release of ChatGPT that did not have the new restrictions. Thirdly, whilst using AI tools, uninformed employees have unintentionally breached Confidentiality Policies and released highly sensitive information. The current “poster boy” for AI based data security breaches is Samsung. In 3 separate incidents this year employees, acting in good faith, have asked ChatGPT to assist with coding projects, but in prompting the bot they released large volumes of very valuable, highly confidential coding to the world. In one example an employee asked ChatGPT to optimize a test sequence process being used to identify faults in Samsung's microchips. By uploading the sequence to ChatGPT the employee released what was a highly confidential process that represented massive IP value to the world. Risk Management neatly summarizes the dilemma: “Most companies have been mishandling data and IT security for years. Rushing to adopt AI technologies on an enterprise-wide scale has just exposed those weaknesses further.” | AI is not uniquely susceptible to security risks, but the recent incidents have highlighted the potential dangers of staff using software when the business does not have:
Action Items:
![]() |
3. Intellectual Property Rights | 3. Intellectual Property Rights |
Current generative AI models operate by scraping massive amounts of data from the internet. Those tools seemingly ignore what are the sources of the information that they use, who owns that information or whether that information is protected by copyright or trademark law. Further, when producing outputs, generative AI tools simply give the user whatever answer it thinks is the best response to the prompt. ChatGPT for example, does not typically provide citations/source attributions unless prompted, and even when citations are asked for it will often simply “make them up”. Additionally, the fundamental issue of “Who owns the IP rights of AI-generated outputs?” still needs to be solved, adding complexity to an already fraught legal landscape. Key questions currently being asked are:
“In other words, it is a plagiarism timebomb from ACME products waiting to explode on the unsuspecting coyote, i.e., you” - Sterling Miller This is not a hypothetical risk, Getty Images is currently suing Stable Diffusion over alleged copyright violation for using their watermarked photo collection. | When you take stuff from one writer it's plagiarism, but when you take from many writers it's called research.” - Wilson Mizner IP agencies, including WIPO, UKIPO, the European Patent Office (“EPO”), the USPTO and the U.S. Copyright Office are scrambling to investigate and set guidelines for many AI‑related IP issues. This includes questions of AI "inventorship", patent eligibility, written description and enablement requirements, data issues, and AI‑related copyright. In the interim, in-house lawyers should appropriately caution business units as to the current uncertainties and risks associated with AI IP. (See Corporate Policies below)
Action Items:
![]() |
4. Ethics, Biases & Blind Spots | 4. Ethics, Biases & Blind Spots |
AI models “reflect” any biases that are incorporated into their programming or the datasets that they are trained on (i.e. the content of the internet). As a result, it, like any software, AI risks discrimination and biases. This risk is particularly acute with AI as lay-users have a tendency to assume that its outputs are “robotic, so must be objective truth free from bias.” The AI models, such as ChatGPT that are also “foundation models” i.e. the infrastructure upon which other AI tools are being built, risk spreading these biases incredibly far and incredibly quickly as downstream AI tools are built upon them. Insider recently demonstrated the strength of these biases by prompting an AI image generator to create a series of images using specific promptly. “American person” resulted in the lightening of the image of a black man, “African worker” resulted in images of gaunt individuals in ragged clothing and primitive tools, whilst “European worker” output images of happy, affluent individuals. These design issues have already led to a number of very real “real world” outcomes for the victims of the bias. For example, the AI system COMPAS (the “Correctional Offender Management Profiling for Alternative Sanctions”) was an algorithm used in US court systems to predict the likelihood that a defendant would become a recidivist. Due to the data and model that COMPAS was based on, it immediately started predicted twice as many false positives for the recidivism of black offenders (45%) than white offenders (23%). Similar issues have been uncovered in systems used by health-care providers and Apple’s HR systems. Additionally, “Toxic Content” such as profanities, identity attacks, sexually explicit content, demeaning language, or incitements to violence have riddled social media platforms for some time. Generative models that mirror language from the web run the risk of propagating such toxicity. See TowardDataScience for further information. Finally, it must be remembered that AI also reflects the “blind spots” inherent to the current version of the internet/dataset that is trained upon. For in-house lawyers, this most obviously manifests when it comes to jurisdiction specific legal issues. Questions relating to American law issues are reasonably accurately addressed by ChatGPT, but answers can be quite unreliable when it comes to questions on other jurisdictions. This is because, there is a “token” (i.e. a gigantic data set) for American contracts / American law issues (i.e. SEC filings), but no such publicly available set of legal documents exists in for UAE law, or Indonesian law or South African law etc. etc. etc. “ChatGPT has no ethics. Seriously, it’s just a machine. It has no ability to discern, apply context, recognize when it is making things up, or deal with or express emotion” | In-house counsel will need to play an important role in their business's procurement of AI technologies, and in leading their teams’ use of AI. As the technology evolves and more data is incorporated, AI's utility is expected to grow. However, companies operating internationally must be mindful of AI's current limitations and the regulatory restrictions that apply in different jurisdictions. At present, the best way to address AI's “biases” is for a human IHL to review outputs and adjust as required (whilst being cognizant that i) it will probably not be possible to completely eradicate it from AI systems, and ii) the human will have their own biases). Such human reviews are important, and demands that in-house lawyers continue to exercise their duties of independence and competence (see Professional Conduct below). “Best Practice” for use of AI, or indeed any automated system, in a legal setting is currently understood to mean keeping a “human/lawyer in the loop”. This means that a human should have the authority and responsibility for altering system outputs to overcome errors / biases / blind spots / hallucinations where possible - Indian Journal of Law and Technology Authorities of various jurisdictions are also working quickly to provide guidelines and tools to help address this issue. For example Singapore has already introduced the Model AI Governance Framework, to promote the governance principles of transparency, accountability, fairness, explain-ability, and robustness, through the use of practical guidelines that organisations use to implement AI responsibly. Singapore has also developed the AI Verify testing framework and toolkit as a way for businesses to test, and demonstrate their implementation of trustworthy AI. Action Items:
![]() |
5. A.I. Lies & Makes Mistakes | 5. A.I. Lies & Makes Mistakes |
“ChatGPT answers more than half of software engineering questions incorrectly” Generative AI does not and cannot discern between come up with a “correct answer” or just “the answer the user wants.” Mistakes made by AI have been anthropomorphized as they are often very vivid - these are now commonly known as “hallucinations”. The difficulty is that AI systems present these hallucinations with the same “perfect poker face” that they use to present every answer they give. There is rarely any qualification or noticeable measure of uncertainty. This makes it very difficult to notice when the AI is simply guessing/generating its own “facts”. One example is in the case of Roberto Mata v Avianca when a lawyer had relied on ChatGPT for research purposes that included several non-existent cases. The court held the lawyer accountable and he was fined for submitting phantom cases. Another instance involves a mayor taking legal action against ChatGPT for it incorrectly stating that he was imprisoned for bribery (he had not been!). ChatGPT's disclaimer does acknowledge these risks, and it is for lawyers to ensure that they are not relying solely on ChatGPT information without verifying that it is i) accurate, and ii) up-to-date . AI systems based on large language models are also susceptible to making errors of fact, logic, mathematics, and common sense problem solving. This is because the models are built upon “natural language” – and whilst language often mirrors the world, it does not do so perfectly and these systems do not (yet) have a deep understanding about how the world being described by that language actually works. ![]() | It is important not to impute deceptive intent / maliciousness (or any other emotions) to AI systems. Rather we must keep in mind that these are simply statistical models interpolating data and filling in the gaps with the results of estimated patterns. It is a duty of in-house lawyers to be sceptical and apply their professional independence and judgement, rather than assuming the infallibility of AI or indeed any technology. Action Items:
"One of my favorite things to do is ask ChatGPT to justify its responses. I'll use phrases like "Why do you think that?" or "What evidence supports your answer?" Often, the AI will simply apologize for making stuff up and come back with a new answer. Other times, it might give you some useful information about its reasoning path.” |
6. Professional Conduct | 6. Professional Conduct |
A number of Legal academics have suggested that a lawyer risk breaching their professional codes of conduct if they start excessively deferring to an AI system's generated outputs. This risk is a particularly acute with AI, as the operations and coding of AI systems are so complex that they are effectively “un-auditable” / it is impossible for a human user to ascertain the basis upon which the outputs were generated. In this context, it is worth remembering that most legal regulatory authorities require their solicitors to comply with variations of the following themes - each of which may be impacted by the use of AI:
| It is not an option to simply say “AI threatens my Pro Conduct compliance, so I will avoid it entirely.” In-house lawyers have a duty to perform competently, and in their clients’ best interests. So, arguably, we may be OBLIGED to use AI - if it improves the quality and efficiency of our work! In certain use cases, e.g. large scale document reviews, this becomes particularly pertinent as AI systems are consistently being shown to operate faster and make fewer mistakes than an “human-eyes only review”.
Action Items:
![]() |
7. It will Steal My Job | 7. It will Steal My Job |
Law, whether in private practice or in-house, has traditionally been based on human-guided expertise (and the billable hour…). So there is justifiable apprehension that digitalization in general, and generative AI in particular, may disrupt career prospects and/or replace roles. “If AI can do in 20 seconds a task that would have taken a dozen associates 50 hours each, then why would big firms continue hiring dozens of associates?” - The Economist To put these numbers in context, a partner at a prestigious NYC corporate-law firm recently suggested that there may be a significant decline from today’s partner to associates ration (which is circa 1/7) to closer to 1/1 at the top firms. ![]() | “Will AI steal my job as an in-house lawyer? Highly unlikely. But It may change it – a lot” – Sterling Miller
AI is a tool that you can use to streamline tasks and reduce the amount of mundane work you must deal with. In particular, by using AI to manage repetitive tasks, in-house lawyers may be able to free up their capacity so that they can focus on higher value, more strategic roles that rely on the exercise of their expertise and experience. The Harvard Business Review recently captured what is generally considered to be “best practice” at the current stage of technological development. Specifically, they argue that despite its recent developments, AI has not, and may never, reach the point that its role is to replace human judgment, but rather its role is to help lawyers to solve problems more efficiently. For example, using AI to quickly identify key legal concepts in contracts, or to analyse historical performance data, allows legal teams to make better informed decisions faster. But there is still a need for a human lawyer to decide how to best use that data to progress the interests of the company. That being said, it would be sensible to take these developments in the legal industry seriously, and proactively ensure that you are “ahead of the curve.” Action Items:
|
8. Corporate Policies | 8. Corporate Policies |
"It is the wild west out there at the moment. many companies (and legal departments) have been caught off-guard by ChatGPT and its popularity" - Risk Management A key tool for risk management within a business, continues to be the policy infrastructure implemented by the business’s in-house legal team. In that context, the UK Law Society recently reported that whilst many businesses have implemented AI guidelines/policies, most of those businesses have only adopted very rudimentary, and typically restrictive, policies. “80% of in-house lawyers that I spoke to work at organisations that have either restricted or blocked access to ChatGPT.” It has also been reported several very large, ostensibly “tech-enabled” companies, such as Amazon, Apple and Verizon have banned all employees from using ChatGPT, whilst JPMorgan Chase, Bank of America and Citigroup have also curtailed its use. Such a “Pull up the drawbridge!” approach is perhaps to be expected from a traditionally very conservative legal industry. However, as mentioned above, such an approach risks inhibiting lawyers from gaining competency with the new technology. Moreover, it has also become very readily apparent that such restrictions are generally being circumvented by staff. Most people appear to be simply ignoring their employer's policies and using ChatGPT for work from their personal devices.
![]() | Generative AI is an area where in-house legal teams can really demonstrate their value to their business colleagues. Policies and procedures put in place to facilitate the implementation of AI, whilst protecting the business and ensuring that employees are using the tools properly. AI Policies based on simply prohibiting AI usage are not an intelligent or effective response to this challenge. Such prohibitions are i) are very easy to circumvent, ii) are very hard to investigate or enforce, and iii) are likely to be counter-productive to the long term growth of the company and its personnel. “AI tools make employees exponentially more productive, and productivity is directly correlated to compensation in the workplace. Companies have been battling shadow IT for 20 years—we do not want a ‘rinse and repeat’ situation with AI tools becoming shadow AI.” - Greg Hatcher (Co-Founder of cybersecurity consultancy White Knight Labs) With this in mind, implementing robust training programs that provide real-world examples to employees is likely to more effectively and more consistently secure a company’s IT ecosystem than trying to impose a simple “AI Prohibition.” Moreover, establishing AI data governance policies is not an impossibly difficult task. “Best Practices” for cybersecurity and control infrastructure have existed and been readily available for years. The current task for in-house lawyers is simply to update and calibrate those existing themes to the new tools. You do not need to create an all-encompassing “AI policy” from scratch. Action Items:
|
9. What Should I use It For? | 9. What Should I use It For? |
The AI models currently available have exhibited emergent capabilities that were far beyond what was expected from their construction. GPT-3 already has 175 billion parameters and the AI models based upon its infrastructure can be quickly adapted to new bespoke tasks, by non-experts/without any coding knowledge, by simply providing it with natural language prompts. So we are all currently in the “experimental stage” of generative AI adoption. Businesses and legal departments alike are conscious that they will need to start using AI somehow, but what exactly are the best “use cases” for AI has not been settled yet. The AI tools on the market today have proven to be surprisingly good at a wide range of tasks that were not necessarily contemplated when the tools were being developed.
![]() |
At this stage, the technology appears to be so adaptable that different businesses, and different units within each business, may end up using AI to solve entirely bespoke challenges. There is not currently a one-size-fits all “use case” for AI. That being said, these tools seem to be particularly effective when it comes to addressing tasks that require huge volumes or data, or many iterations of repetitive tasks. For example, the Australian Government Productivity Commission reported that the Commonwealth Bank of Australia and ING used AI to interpret about 1.5 million paragraphs of regulation on the European Union’s Markets in the Financial Instruments Directive. Manually, this task would have taken circa 1,800 man-hours (or one year’s work for one full-time employee) to complete. But the use of AI enabled the bank to complete the task in two and a half minutes. See the section “AI TOOLS AVAILABLE FOR IN-HOUSE LAWYERS TODAY” for specific examples of currently available AI tools and their uses. However, AI is most quickly being adopted by in-house teams to:
When adopting and implementing AI, legal teams should however carefully plan and develop a digital roadmap to avoid fragmented technology implementations. Instead of buying multi-point solutions, they should align their digital workflow and data with their business's objectives, considering AI as part of a holistic strategy. Integrating various AI systems and technologies is crucial for seamless communication and overall efficiency. Action Items:
|
10. What Skills Do I Need to Use AI Well? | 10. What Skills Do I Need to Use AI Well? |
AI, and in particular AI tools based on the ChatGPT architecture, are driving a new skillset requirement for in-house lawyers. These tools use natural language inputs or “Prompts” as their control mechanism. Like any skill, it takes some time and practice to learn how to prompt well/effectively/efficiently. Currently everyone is basically operating at a “beginner” level, but the world is quickly dividing into the “skilled prompters” and the “kooks.”
![]() | Say that you want to draft an indemnity clause. You could simply ask ChatGPT to “Draft an indemnity.” From this you will get a reasonable answer. However, you' will get a far more nuanced answer if you apply each of these “best practices” in order:
Contract Nerds provides an excellent working example of how in-house lawyers can Prime, Prompt, Refine, Iterate and Probe ChatGPT to address real world contracting tasks. Action Items:
|
CONCLUSION
The role of in-house counsel has become more multi-faceted, requiring skills in management and procurement and legal operations AND the ability to supervise both staff and technology effectively.
The typical in-house lawyer could happily work the rest of their career with only a passing awareness of technology “fads” like NFTs. However, lawyers and the in-house legal departments that fail to get a working understanding of AI may in the relatively near future come to be seen as operating inexcusably inefficiently.
The good news is that there are lots of tools, guidance and policy resources readily available (often for free) to those in-house lawyers who are willing to make the most of them.
AI TOOLS IN-HOUSE LAWYERS CAN USE TODAY
Sterling Miller has been incredibly helpful for the in-house legal community and consolidated together a list of some of the day-to-day tasks that AI can be used by in-house lawyers today. His list is as follows:
- E-Discovery document review (e.g., DISCO Cecilia)
- Legal research (ChatGPT or Alexsi)
- Draft memoranda (ChatGPT)
- Draft email (ChatGPT)
- Predict litigation outcomes (Lex Machina or Solomonic)
- Draft legal briefs and motions (Lexis+ or ChatGPT)
- Create clipart (Midjourney)
- M&A due diligence (Ansarada or Kira)
- Summarize articles/documents (ChatGPT)
- Translate into your first language (ChatGPT)
- Transcribe meetings/calls (ChatGPT or Otter.ai or Fireflies.ai) [9]
- Prepare slides (ChatGPT)
- Jury research (ChatGPT)
- Legal brainstorming (ChatGPT)
- Create a chatbot for frequently asked questions (Josef, Clio, Poe)
- Review legal and vendor invoices (Brightflag or Onit)
- Simplify text (ChatGPT)
- Create checklists (ChatGPT)
- Redline documents (BlackBoiler)
- Responding to redlines (see Chatting Contracts examples above in No. 4)
- Contract drafting and analysis (Spellbook or Kira)
- Edit your writing (Grammarly or Briefcatch)
- Deposition prep kits (ChatGPT or aiLawDocs)
- Negotiate edits to contracts/align contracts to playbooks (DocJuris)
Ready To Transform Your Legal Team?
Please check out the GLS solutions and know-how resources listed on the right side of this page – they might assist your legal team with the issues explored in this Blog.
© The GLS Group - Law Rewritten
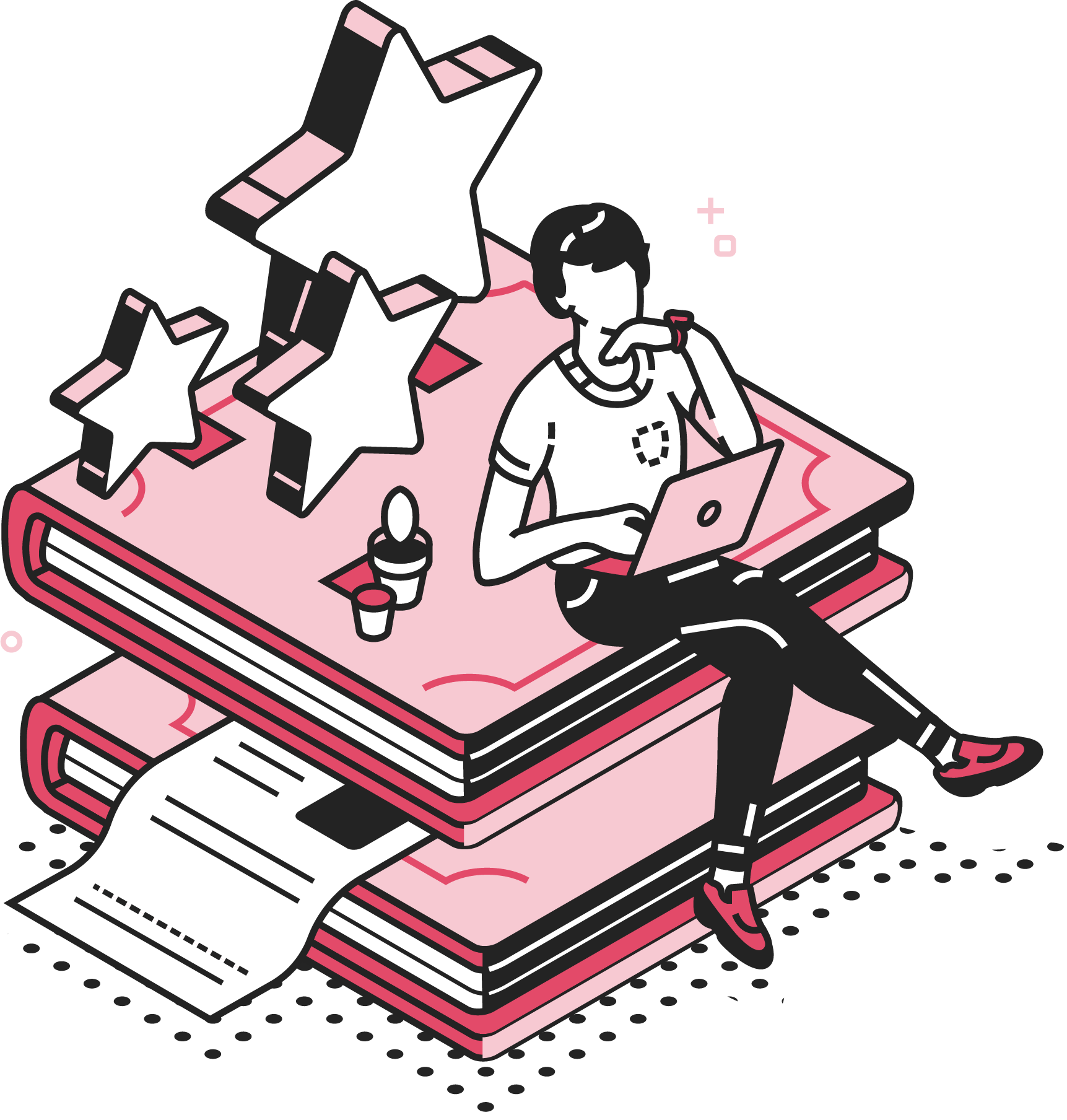
The GLS Legal Operations Centre
Register to access your complimentary Day 1 Resource Stack packed with legal team performance resources.
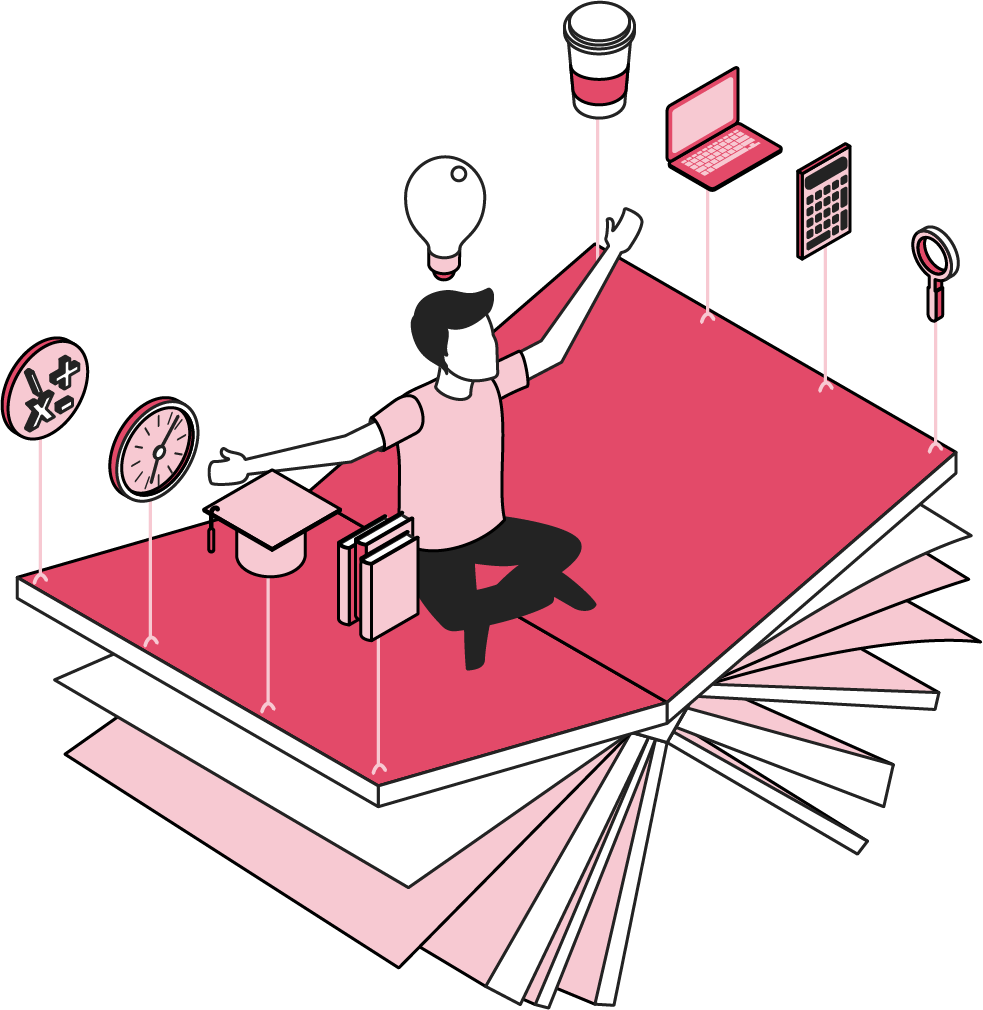
GLS Ultimate Guide To Legal Operations
Download this and read it thoroughly and regularly. It is a wonderful transformation companion.
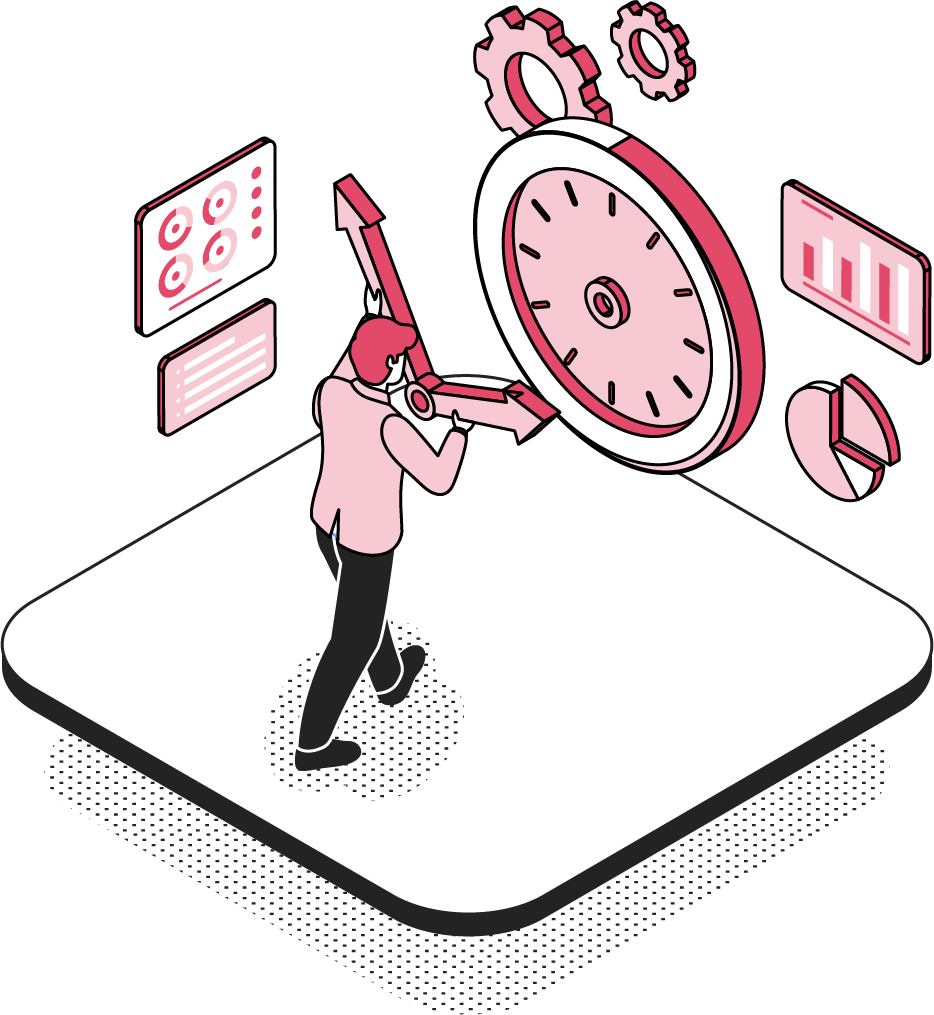
Book A No-Obligation Consultation
If you would like discuss your legal transformation needs, please book a 30 minute free consultation with us.
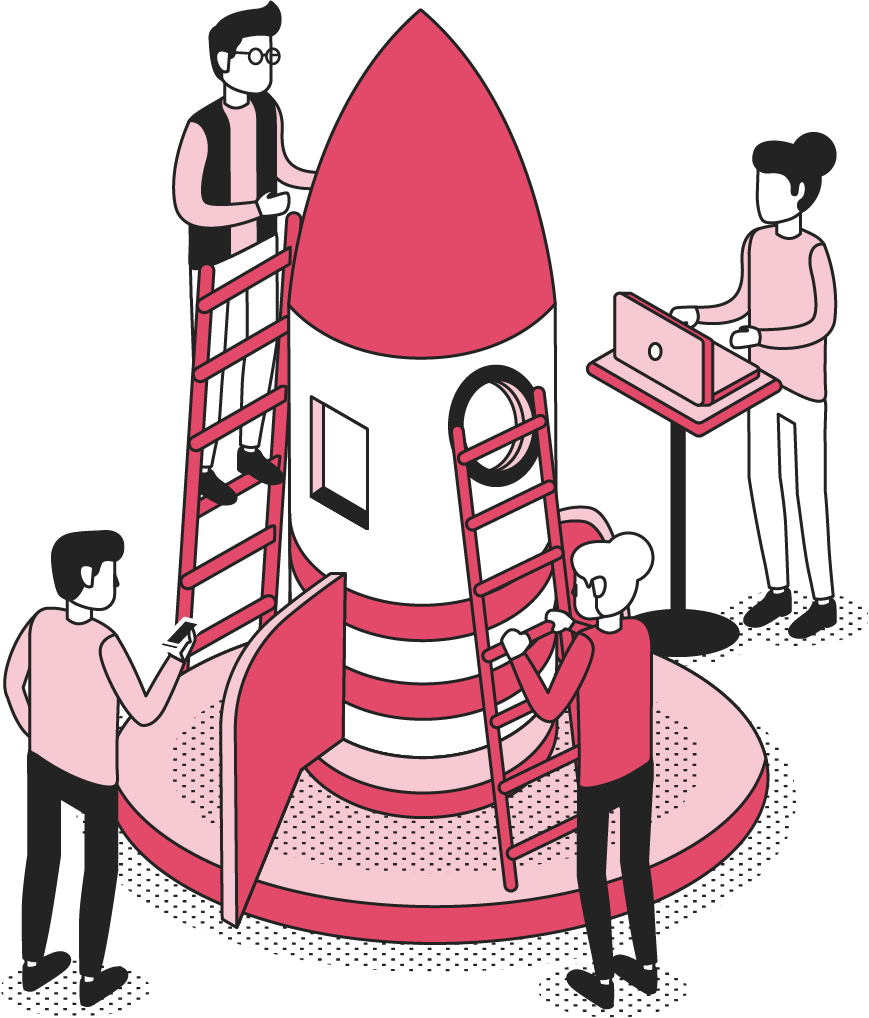
GLS Legal Transformation Boot Camp
Our hugely successful, 10-week long, email-based boot camp on how to effectively transform your legal team.